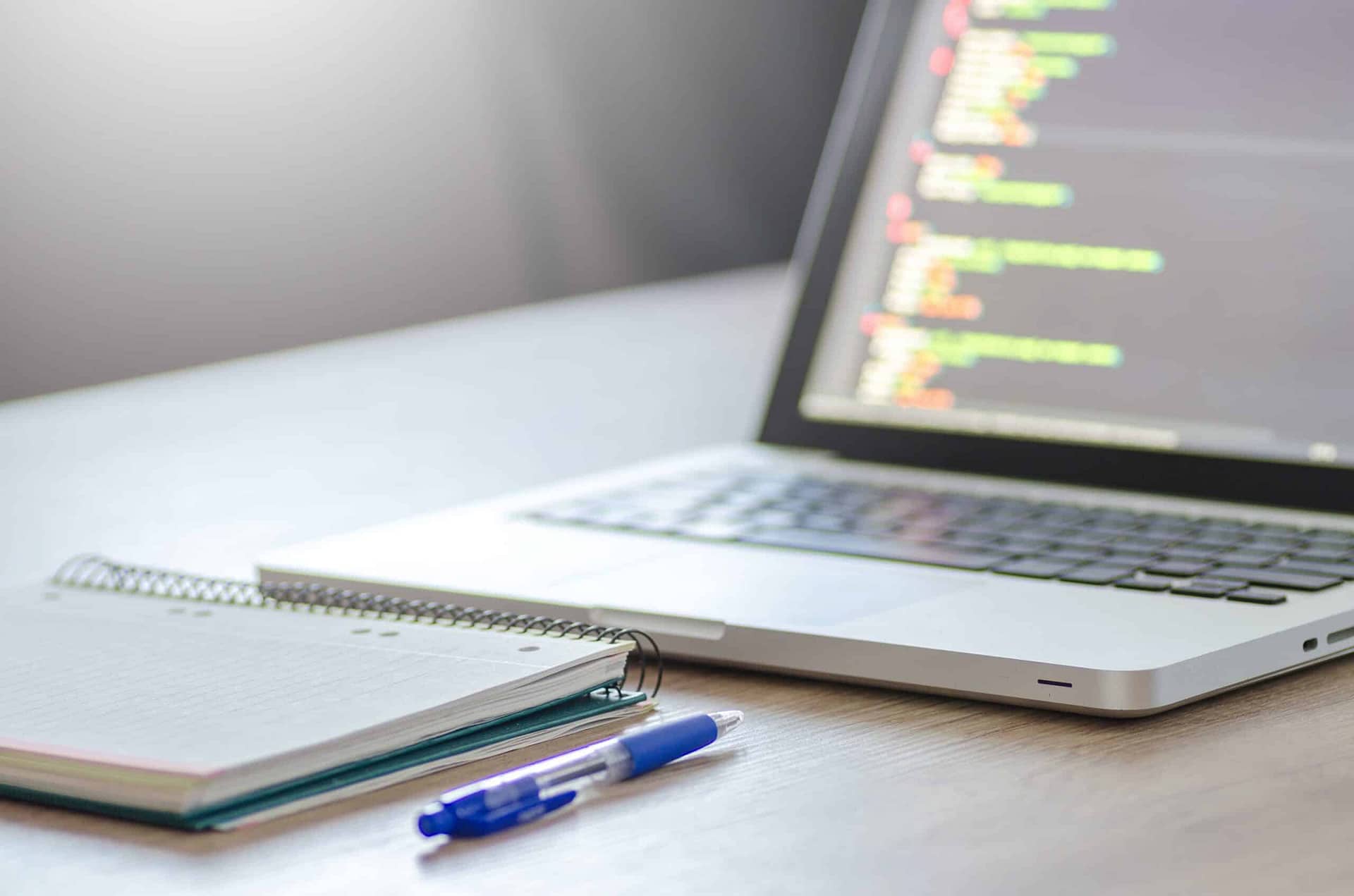
Applications of Data Science in Pricing
Beyond the “Mission Critical” character of defining a relevant pricing strategy, it’s now a question of optimizing its execution, and it’s on this point that Data Science has become an essential asset for retailers. It enables them to analyze, predict and prescribe the best actions to optimize prices for each product cluster and consumer segment.
The ambition is simple: to create tangible, relevant and sustainable value over time!
In order to do so, your data needs to be easily accessible, reliable and granular. Without these prerequisites, you risk errors and biases when using AI in your pricing strategies. Thus, to implement pricing optimization, we recommend you to have access to your product catalog data flow – this is the very basis – as well as additional data flows such as: transactional data, competition data, traffic data, etc.
Now, explore with us the four great missions of Data Science for pricing, as well as the right time to involve Data Science experts and the best approach to convince your audience.
The 4 major missions of Data Science for Pricing
LEARN
This mission involves understanding consumer sensitivity to pricing in different contexts, product clusters and consumer segments. This involves considering the impact of internal (e.g. marketing campaigns) and external (e.g. competitors) events on various financial and behavioral KPIs, precisely in order to isolate the impact of pricing actions.
The algorithmic challenge lies in the reliability of price elasticity learning. This mission of constant learning is a complex one, as it requires not only isolating the impact of the pricing action in view of the current context, but also maximizing the targeted performance and respecting the defined strategic and/or operational differences.
This mission is not only algorithmic, but also ergonomic, as the ultimate ambition can only be achieved if the user’s trust is obtained. Thus, there is a challenge to popularize the learning acquired in terms of price sensitivity by cluster, segment, context and on each of the relevant KPIs… Indeed, it is essential to make the results of the analysis accessible and comprehensible to each of the businesses involved in the decision-making and implementation process.
PREDICT
Anticipating the impact of actions on prices is a major challenge, hence the importance of quality learning.
This prediction exercise is an essential step in the quest to define the optimal price level. Indeed, we now need to put our learning to the test by exploring multiple scenarios (e.g. combination of price variation by product cluster and consumer segment) in order to predict their respective impacts on each of the relevant KPIs.
Obviously, this prediction is made with the consideration of an error rate or confidence index, in an attempt to maximize the reliability of the expected impact of each scenario.
Finally, pricing is a field where the famous saying “today’s truth is not tomorrow’s” is particularly relevant. It is therefore not only necessary to learn elasticity constantly, via reinforcement learning, but also to constantly predict the impact of each scenario. Indeed, the nature of elasticity, being in many cases not only non-linear and non-identical between clusters or segments, but also non-constant over time, implies a reassessment of the expected impact of the scenarios.
PRESCRIBE
Prescribing consists in taking advantage of the 2 previous stages, learning and prediction, to aim for the ultimate ambition of relevant optimization. This involves suggesting price change actions that:
- Respect financial constraints (e.g. a minimum average margin rate or minimum performance on a given KPI)
- AND respect operational and strategic constraints (e.g. frequency or size of price changes)
- AND maximize the performance of the KPI to be optimized and the KPIs to be protected.
As consumers’ price sensitivity evolves, so must these recommendations, to ensure consistency between recommendation and contextual market reality. Today’s optimal price suggestion is not necessarily tomorrow’s reality.
Price recommendations must be as transparent as possible, to facilitate arbitration when making decisions.
MEASURE
Quantifying the isolated impact of pricing, despite its cross-company nature, is a challenge for data science. This mission is a real balancing act, because :
- Reliability requires the use of algorithmic models to eliminate contextual effects (internal and external events)
- Transparency requires simplicity to enable reproducibility.
At PricingHUB, we have developed several methods for measuring value creation, in order to validate with the customer the one most relevant to his or her case, explaining the pros and cons of each and achieving the trust. To find out more, contact us here.
When should I call on a Data Science resource?
In our view, Data Science can be brought into the pricing process at several key moments:
- Convince: It can help persuade decision-makers by streamlining the approach and bringing confidence to pricing recommendations.
- Build: Data Science experts develop algorithmic features to create relevant value.
- Improve: Reducing the error rate of learning is an infinite exercise, as perfect knowledge of price sensitivity is unattainable, but essential as it is the foundation of optimization on which the whole process rests.
- Plan the vision: It’s essential to segment improvement and construction actions by stage, to execute them progressively, and to deliver the added value of each stage.
What’s the right format for convincing employees?
There’s no magic formula.
The consensus is clearly that avoiding the black box effect and simplifying complex concepts as much as possible for a non-technical audience is a complex exercise. Nevertheless, meeting the challenges of competence, popularization and time is essential to gain trust and buy-in for the project internally as soon as possible.
Business realities often demand rapid trust, which can make the equation even more complex. However, by establishing an open dialogue with stakeholders, explaining the methods and demonstrating the long-term benefits, it is possible to convince your audience more easily.
The challenges of data science in pricing continue to grow, with a constant need for adaptation and innovation to meet changing market demands.
Data science in pricing is not just a tool, but a catalyst for transformation, offering constantly renewed opportunities to optimize, predict, prescribe and measure, in order to guide companies’ strategic and operational decisions.
The future of this synergy between data science and pricing is promising, offering opportunities for continuous optimization and informed decision-making for market players.