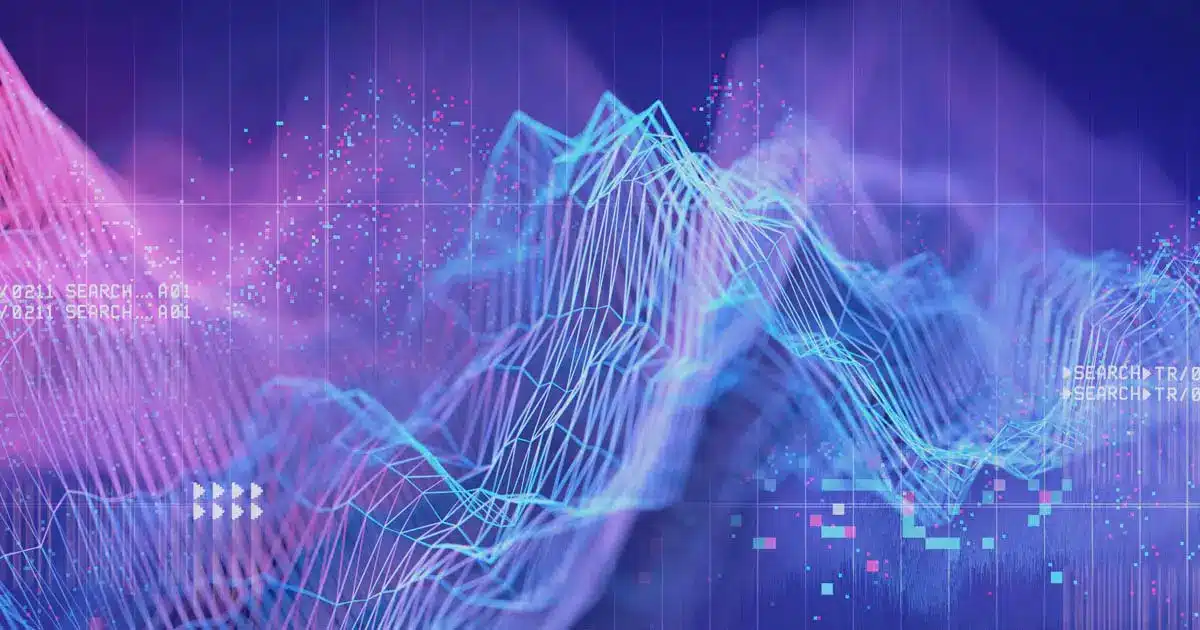
Driving price elasticity with machine learning
Xavier Casellato, co-founder of PricingHUB was sharing with Télécom Paris students as part of the MS Big Data and IA seminars our data science approach. Interesting debates around why we prefer to use adaptive rather than a predictive model when it comes to computing price elasticity.
Where a lot of effort from resellers is directed at generating traffic, converting visitors to their platform and boosting sales, PricingHUB has chosen instead to focus on price elasticity in order to achieve an optimal profit margin.
For each client, PricingHUB builds a product elasticity curve and seeks to understand the impact of price changes on sales volumes, turnover and margin mass. The solution is based on a machine learning algorithm and is based on a generic model that will then be specified: each client can define business rules, constraints, such as minimum margin rates in case they have incompressible fixed costs, for example.
The advantage of an adaptive rather than predictive model
The models are based on the understanding of price elasticity at a specific point in time and in a global context (e.g. competition, seasonality, external and/or internal events affecting purchasing behaviour…). To achieve this, PricingHUB has developed its model with reinforcement learning techniques that constantly test prices and measure performance against business objectives.
Reinforcement learning algorithms calculate a price recommendation adjusted each time for the next iteration. This method allows to manage unexpected and unpredictable edge effects, as opposed to predictive models that are exposed to a lot of noise.
Today, retailing and more specifically e-commerce has entered a zone of instability. Conventional predictive models will be less effective in accounting for this phenomenon, where adaptive models are particularly relevant in uncertain environments.
Also, PricingHUB has an ambitious roadmap in terms of:
- Enhancements to maximize the adoption of the platform among its users,
- Increasing the quality of learning about contextual elasticity in order to be more relevant and value-creating in the price recommendations issued,
- Creation of new use cases to accelerate the effects of elasticity (stock, merchandising, cross-sell…),
- Automation and scalability of all the processes & models that consume the most system resources.
You can read the full article by clicking here!